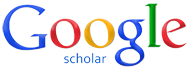


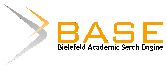


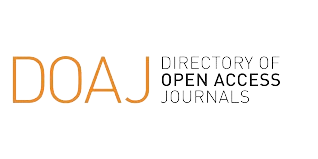
Diterbitkan Oleh:
Lembaga Penelitian Pengabdian Masyarakat Universitas Nusa Mandiri
Creation is distributed below Lisensi Creative Commons Atribusi-NonKomersial 4.0 Internasional.
The drop-out student is a student whose student status is revoked, in case of determined orders by the university. The height of drop-out students number will have an impact on the scoring of accreditation in a university. therefore, it is necessary to know what factors the students have the drop-out status are. Most of the algorithm comparison has been done in previous research. Otherwise, this research uses Neural Network with Particle Swarm Optimization based model to level up optimation value in selection attribute influencing prediction the students drop out.
An author who publishes in the Pilar Nusa Mandiri: Journal of Computing and Information System agrees to the following terms:
Diterbitkan Oleh:
Lembaga Penelitian Pengabdian Masyarakat Universitas Nusa Mandiri
Creation is distributed below Lisensi Creative Commons Atribusi-NonKomersial 4.0 Internasional.