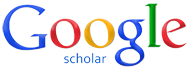


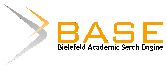


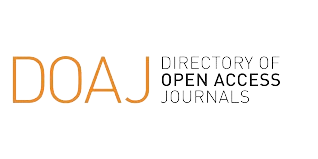
Diterbitkan Oleh:
Lembaga Penelitian Pengabdian Masyarakat Universitas Nusa Mandiri
Creation is distributed below Lisensi Creative Commons Atribusi-NonKomersial 4.0 Internasional.
Every year college get scholarship coordinator private universities (kopertis), Scholarship devoted to student in a college namely scholarship an increase in academic performance (scholarship PPA ) and scholarship assistance learn student (scholarship BBM). The process filing scholarship ppa and bbm through two stages selection selection is the first stage selection in college to determine a candidate scholarship recipients that would be proposed to kopertis, the selection that both the stage in kopertis selection. Many a student who submitted the scholarships as well as surpassing kouta given resulted in the process of select recipients taken longer since select must be in accordance with the criteria so that the recipient scholarship right on target . Based on these problems need a the act of determining scholarship recipients proper. The purpose of this research is to make classifications students scholarship recipients with algorithm C4.5. The results of classifications evaluate and validated with confusion matrix and a curve ROC, the results classifications students scholarship recipients namely algorithm C4.5 with the level of accuracy of 86.88 %, So that it can be applied for the problem the determination of scholarship recipients .
Gorunescu, F. 2011. Data Mining Concepts, Models and Techniques. Berlin: Springer.
Han, J., & Kamber, M. 2006. Data Mining Concepts And Techniques 2nd Edition. San Fransisco: Elsevier.
Jones, P. 2001. Visual Basic: A Complete Course. London: Continuum.
Karismariyanti, M. 2011. Simulasi Pendukung Keputusan Penerima Beasiswa Menggunakan Metode Composite Performance Index. Jurnal Teknologi Informasi Vol.1 , 54-59.
Kotsiantis, S., & Pintelas. P. 2004. A Decision Support Prototype Tool for Predicting Student Performance in an ODL Environment. Greece: University of Patras.
Larose, D. T. 2005. Discovering Knowledge in Data An Introduction to Data Mining. New Jersey: John Willey and Sons.
Liao, T. W. 2007. Enterprise Data Mining: A Review and Research Directions. Recent Advances in Data Mining of Enterprise Data: Algorithms and Applications , 1-109.
Liberty, J. 2005. Programming Visual Basic 2005. USA: O'Reilly Media.
Maimon, O., & Rokach, L. 2010. Data Mining and Knowledge Discovery Handbook Second Edition. London: Springer.
Powers, D. 2011. Evaluation: From Precision, Recall and F-Measure To ROC, Informedness, Markedness & Correlation. Journal of Machine
Learning Technologies , 37-63.
Santiary, P. A. 2012. Sistem Pendukung Keputusan Cerdas dalam Penentuan Beasiswa. Jurnal Logic Vol.12 No.2 , 87-91.
Suryana, T. 2009. Visual Basic. Yogyakarta: Graha Ilmu.
Vercellis, C. 2009. Business Intelligence. United Kingdom: John Wiley and Sons.
Witten, I. H., Frank, E., & Hall, M. A. 2011. Data Mining Practical Machine Learning Tools and Techniques Third Edition. Burlington: Elsevier.
Wu, X., & Kumar, V. 2009. The Top Ten Algorithms in Data Mining. USA: CRC Press.
www.dikti.go.id/.januari-13,2014.
An author who publishes in the Pilar Nusa Mandiri: Journal of Computing and Information System agrees to the following terms:
Diterbitkan Oleh:
Lembaga Penelitian Pengabdian Masyarakat Universitas Nusa Mandiri
Creation is distributed below Lisensi Creative Commons Atribusi-NonKomersial 4.0 Internasional.