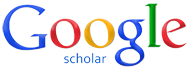


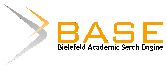


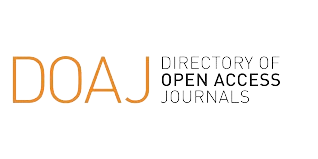
Diterbitkan Oleh:
Lembaga Penelitian Pengabdian Masyarakat Universitas Nusa Mandiri
Creation is distributed below Lisensi Creative Commons Atribusi-NonKomersial 4.0 Internasional.
Lombok is attracted to the Moto GP event, which is held annually. Various tourism brands are owned by the island of Lombok, one of which is Mutiara. The ideal Pearl is perfectly round and smooth, but there are a variety of other shapes as well. One method that can be used to process Pearl's image is Computer Vision. For that, it is necessary to have a way to classify the quality of a Pearl based on its shape. The purpose of this study is to propose a system for pearl image classification by combining feature extraction with artificial neural networks. The method used in this study is GLCM feature extraction and Neural Networks. The proposed system can provide good classification results by combining the GLCM method and the Neural Network. This study uses Epochs 5, 10, 15, 30, 50, 100, 200, 300, and 500 with a learning rate of 0.5. The results of this study indicate that Epoch 100 gives the highest accuracy, 91.66%.
An author who publishes in the Pilar Nusa Mandiri: Journal of Computing and Information System agrees to the following terms:
Diterbitkan Oleh:
Lembaga Penelitian Pengabdian Masyarakat Universitas Nusa Mandiri
Creation is distributed below Lisensi Creative Commons Atribusi-NonKomersial 4.0 Internasional.